[1]:
# To run this in Google Colab, uncomment the following line
# !pip install geometric_kernels
# If you want to use a version of the library from a specific branch on GitHub,
# say, from the "devel" branch, uncomment the line below instead
# !pip install "git+https://github.com/geometric-kernels/GeometricKernels@devel"
Gaussian Process Regression on a Mesh with GPJax¶
This notebooks shows how to fit a GPJax Gaussian process (GP) on a mesh.
This notebook is written for GPJax 0.6.9 which requires tensorflow-probability<0.20.0, which requires tensorflow 2.11, which does not support mac-arm64. Because of this mess with dependencies, we do not test this notebook in our workflows. Hopefully, this will change in the future. Note: actually, GPJax 0.6.9 seems to run fine with tensorflow-probability-0.23 and tensorflow-2.15, if you are willing to force-install them breaking the formal requirements.
[1]:
# Import a backend, we use jax in this example.
import jax.numpy as jnp
import jax
import gpjax as gpx
# Import the geometric_kernels backend.
import geometric_kernels
import geometric_kernels.jax
# Import the Mesh space and the general-purpose MaternGeometricKernel
from geometric_kernels.spaces.mesh import Mesh
from geometric_kernels.kernels import MaternGeometricKernel
# The GPJax frontend of GeometricKernels
from geometric_kernels.frontends.gpjax import GPJaxGeometricKernel
# Sampling routines we will use to create a dummy dataset
from geometric_kernels.kernels import default_feature_map
from geometric_kernels.sampling import sampler
from geometric_kernels.utils.utils import make_deterministic
# Stuff
import numpy as np
import optax
import plotly.graph_objects as go
from plotly.subplots import make_subplots
from pathlib import Path
jax.config.update("jax_enable_x64", True)
INFO (geometric_kernels): Numpy backend is enabled, as always. To enable other backends, don't forget `import geometric_kernels.*backend name*`.
INFO (geometric_kernels): JAX backend enabled.
Mesh Plotting Utils for plotly
¶
[2]:
def update_figure(fig):
"""Utility to clean up figure"""
fig.update_layout(scene_aspectmode="cube")
fig.update_scenes(xaxis_visible=False, yaxis_visible=False, zaxis_visible=False)
# fig.update_traces(showscale=False, hoverinfo="none")
fig.update_layout(margin=dict(l=0, r=0, t=0, b=0))
fig.update_layout(plot_bgcolor="rgba(0,0,0,0)", paper_bgcolor="rgba(0,0,0,0)")
fig.update_layout(
scene=dict(
xaxis=dict(showbackground=False, showticklabels=False, visible=False),
yaxis=dict(showbackground=False, showticklabels=False, visible=False),
zaxis=dict(showbackground=False, showticklabels=False, visible=False),
)
)
return fig
def plot_mesh(mesh: Mesh, vertices_colors = None, **kwargs):
plot = go.Mesh3d(
x=mesh.vertices[:, 0],
y=mesh.vertices[:, 1],
z=mesh.vertices[:, 2],
i=mesh.faces[:, 0],
j=mesh.faces[:, 1],
k=mesh.faces[:, 2],
intensity=vertices_colors,
**kwargs
)
return plot
Defining a Space¶
First, we create a GeometricKernels space that corresponds to a teddy bear mesh loaded from “../data/teddy.obj”
[3]:
mesh = Mesh.load_mesh(str(Path.cwd().parent / "data" / "teddy.obj"))
print("Number of vertices in the mesh:", mesh.num_vertices)
Number of vertices in the mesh: 1598
Now we actually visualize the mesh.
[4]:
# Define the camera
camera = dict(
up=dict(x=0, y=1, z=0),
center=dict(x=0, y=0, z=0),
eye=dict(x=0, y=0.7, z=1.25)
)
plot = plot_mesh(mesh)
fig = go.Figure(plot)
update_figure(fig)
fig.update_layout(
scene_camera=camera
)
fig.show("png")
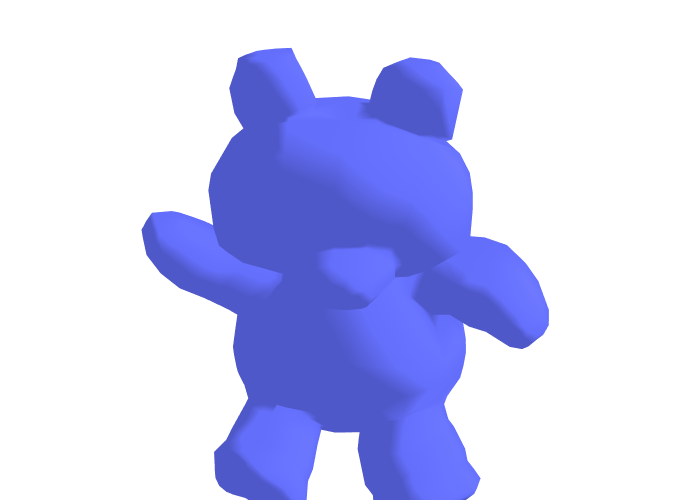
Create a Dummy Dataset on the Mesh¶
We sample from the prior of a GP to create a simple dataset we can afterwards fit using an exact Gaussian process regression (GPR) model.
The input set \(X \in \mathbb{N}^{n \times 1}\) consists of indices enumerating vertices of the mesh. Consequently, the elements of \(X\) are in \([0, N_v-1]\), where \(N_v\) are the number of vertices in the mesh. We sample num_data
of them into the tensor called xs_train
. For test inputs xs_test
, we use the whole \(X\).
[5]:
num_data = 50
key = jax.random.PRNGKey(1234)
xs_train = jax.random.randint(key, minval=0, maxval=mesh.num_vertices, shape=(num_data, 1), dtype=jnp.int64)
xs_test = jnp.arange(mesh.num_vertices, dtype=jnp.int64)[:, None]
# print("xs_train:", xs_train)
# print("xs_test:", xs_test)
To generate the corresponding outputs ys_train
and ys_test
, we sample from the prior. To do this, we create a MaternGeometricKernel
object and use the efficent sampling functionality of GeometricKernels.
[6]:
base_kernel = MaternGeometricKernel(mesh)
params = base_kernel.init_params()
params["lengthscale"] = jnp.array([5.0], dtype=jnp.float64)
params["nu"] = jnp.array([2.5], dtype=jnp.float64)
feature_map = default_feature_map(kernel=base_kernel)
sample_paths = make_deterministic(sampler(feature_map), key)
_, ys_train = sample_paths(xs_train, params)
key, ys_test = sample_paths(xs_test, params)
assert(jnp.allclose((ys_test[xs_train[:, 0]]), ys_train))
# print("ys_train:", ys_train)
# print((ys_test[xs_train])[:, :, 0])
Build a GPJax Model¶
Now we wrap the base_kernel
created above into the GPJaxGeometricKernel
to make an actual GPJax kernel.
Note: params
are external to the base_kernel
object, thus we need to pass them to the GPJaxGeometricKernel
explicitly. Otherwise it will use params = base_kernel.init_params()
.
[7]:
kernel = GPJaxGeometricKernel(base_kernel=base_kernel,
nu=params["nu"],
lengthscale=params["lengthscale"],
variance=1.0)
# Uncomment the lines below if you want optimizable nu.
# Note: if nu is initialized to jnp.inf, it will not be optimizable either way.
# kernel = kernel.replace_trainable(nu=True)
# print("Info on Trainable Parameters:", kernel.trainables())
We use the data xs_train
, ys_train
and the GPJax kernel kernel
to construct a GPJax model.
[8]:
data = gpx.Dataset(X=xs_train, y=ys_train)
prior = gpx.Prior(mean_function=gpx.mean_functions.Zero(), kernel=kernel)
likelihood = gpx.likelihoods.Gaussian(num_datapoints=num_data, obs_noise=1e-4)
posterior = likelihood * prior
print("Initial model:")
print("kernel.nu =", prior.kernel.nu)
print("kernel.lengthscale =", prior.kernel.lengthscale)
print("kernel.variance =", prior.kernel.variance)
print("likelihood.obs_noise =", likelihood.obs_noise)
print("")
negative_mll = gpx.objectives.ConjugateMLL(negative=True)
print("Initial negative log marginal likelihood:", negative_mll(posterior, train_data=data))
/Users/vabor112/anaconda3/envs/gkconda_updated_sh/lib/python3.10/site-packages/gpjax/dataset.py:58: UserWarning:
X is not of type float64. Got X.dtype=int64. This may lead to numerical instability.
Initial model:
kernel.nu = [2.5]
kernel.lengthscale = [5.]
kernel.variance = 1.0
likelihood.obs_noise = 0.0001
Initial negative log marginal likelihood: 45.696745747697115
Train the Model (Optimize Hyperparameters)¶
[9]:
negative_mll = jax.jit(negative_mll)
print("Starting training...")
opt_posterior, history = gpx.fit(
model=posterior,
objective=negative_mll,
train_data=data,
optim=optax.sgd(0.01),
key=key,
)
print("Final model:")
print("kernel.nu =", opt_posterior.prior.kernel.nu)
print("kernel.lengthscale =", opt_posterior.prior.kernel.lengthscale)
print("kernel.variance =", opt_posterior.prior.kernel.variance)
print("likelihood.obs_noise =", opt_posterior.likelihood.obs_noise)
print("")
print("Final negative log marginal likelihood:", negative_mll(opt_posterior, train_data=data))
Starting training...
Final model:
kernel.nu = [2.5]
kernel.lengthscale = [4.29801996]
kernel.variance = 0.9459238
likelihood.obs_noise = 3.7371807e-05
Final negative log marginal likelihood: 43.863126607136955
Draw Predictions and Evaluate the Model¶
Recall that xs_test
contains all the locations on the mesh, i.e. all numbers from the set \(\{0, 1, \ldots, N_v-1\}\).
[10]:
# predict mean and variance
latent_dist = opt_posterior.predict(xs_test, train_data=data)
posterior_mean = jnp.reshape(latent_dist.mean(), ys_test.shape)
posterior_std = jnp.reshape(latent_dist.stddev(), ys_test.shape)
# predict sample
sample = latent_dist.sample(seed=key, sample_shape=(1,))[0, :, None]
[11]:
# Mark training data
training_data_coords = mesh.vertices[xs_train[:, 0]]
training_data_plot = go.Scatter3d(
x=np.array(training_data_coords[:, 0]),
y=np.array(training_data_coords[:, 1]),
z=np.array(training_data_coords[:, 2]),
mode = "markers",
marker_color = "red",
name="",
showlegend=False,
)
# Various plots as plotly traces
ground_truth_plot = plot_mesh(mesh, vertices_colors=ys_test, colorscale="Viridis",
colorbar=dict(
x=0,
y=0.75,
xanchor="center",
yanchor="middle",
len=0.4,
thicknessmode="fraction",
thickness=0.01)
)
sample_plot = plot_mesh(mesh, vertices_colors=sample, colorscale="Viridis",
colorbar=dict(
x=1.0,
y=0.75,
xanchor="center",
yanchor="middle",
len=0.4,
thicknessmode="fraction",
thickness=0.01)
)
posterior_mean_plot = plot_mesh(mesh, vertices_colors=posterior_mean, colorscale="Viridis",
colorbar=dict(
x=0.0,
y=0.25,
xanchor="center",
yanchor="middle",
len=0.4,
thicknessmode="fraction",
thickness=0.01)
)
posterior_std_plot = plot_mesh(mesh, vertices_colors=posterior_std, colorscale="Plasma",
colorbar=dict(
x=1.0,
y=0.25,
xanchor="center",
yanchor="middle",
len=0.4,
thicknessmode="fraction",
thickness=0.01)
)
# Setting up the layout
fig = make_subplots(
rows=2, cols=2,
specs=[[{"type": "surface"}, {"type": "surface"}],
[{"type": "surface"}, {"type": "surface"}]],
subplot_titles=(r"Ground Truth",
r"Posterior Sample",
r"Prediction",
r"Posterior Standard Deviation"),
vertical_spacing=0.1)
# Adding the traces
fig.add_trace(ground_truth_plot, row=1, col=1)
fig.add_trace(training_data_plot, row=1, col=2)
fig.add_trace(sample_plot, row=1, col=2)
fig.add_trace(training_data_plot, row=2, col=1)
fig.add_trace(posterior_mean_plot, row=2, col=1)
fig.add_trace(training_data_plot, row=2, col=2)
fig.add_trace(posterior_std_plot, row=2, col=2)
fig = update_figure(fig)
fig.layout.scene1.camera = camera
fig.layout.scene2.camera = camera
fig.layout.scene3.camera = camera
fig.layout.scene4.camera = camera
fig.update_layout(
margin={"t": 50},
)
fig.show("png", width=1000, height=800)
# fig.show()
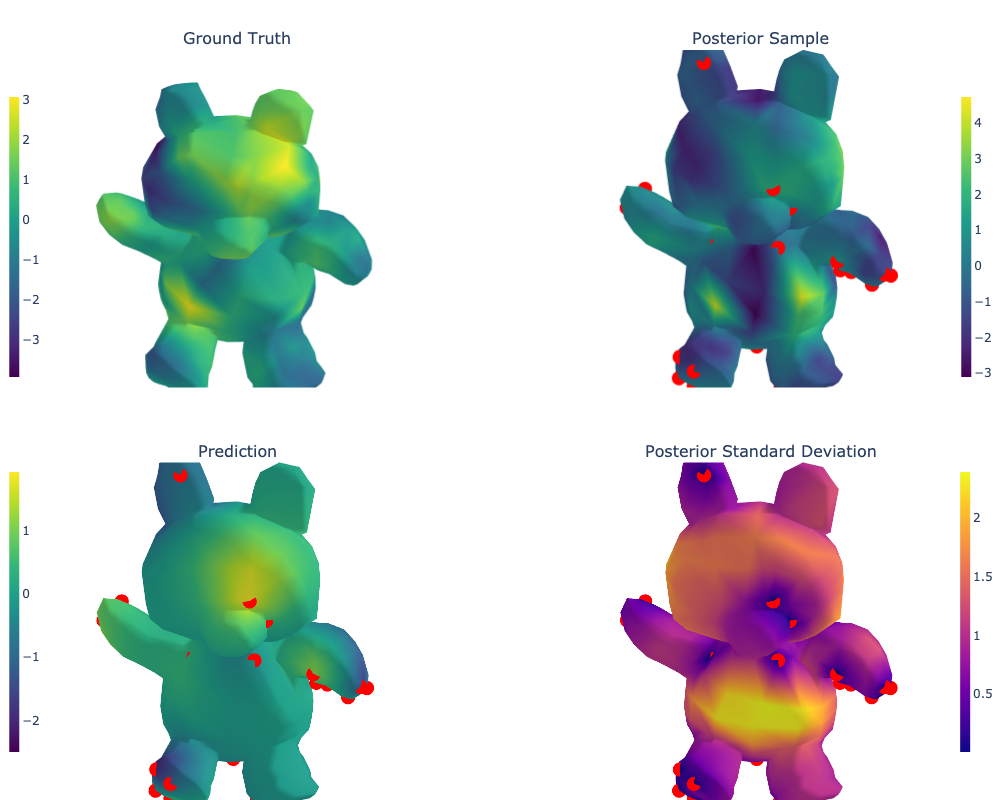
Citations¶
If you are using meshes and GeometricKernels, please consider citing
@article{mostowsky2024,
title = {The GeometricKernels Package: Heat and Matérn Kernels for Geometric Learning on Manifolds, Meshes, and Graphs},
author = {Peter Mostowsky and Vincent Dutordoir and Iskander Azangulov and Noémie Jaquier and Michael John Hutchinson and Aditya Ravuri and Leonel Rozo and Alexander Terenin and Viacheslav Borovitskiy},
year = {2024},
journal = {arXiv:2407.08086},
}
@inproceedings{borovitskiy2020,
title={Matérn Gaussian processes on Riemannian manifolds},
author={Viacheslav Borovitskiy and Alexander Terenin and Peter Mostowsky and Marc Peter Deisenroth},
booktitle={Advances in Neural Information Processing Systems},
year={2020}
}
@article{sharp2020,
author={Nicholas Sharp and Keenan Crane},
title={A Laplacian for Nonmanifold Triangle Meshes},
journal={Computer Graphics Forum (SGP)},
volume={39},
number={5},
year={2020}
}